Manifold
Manifold is a model-agnostic visual debugging tool for machine learning.
Understanding ML model performance and behavior is a non-trivial process, given intrisic opacity of ML algorithms. Performance summary statistics such as AUC, RMSE... are not instructive enough for identifying what went wrong with a model or how to improve it.
As a visual analytics tool, Manifold allows ML practitioners to look beyond overall summary metrics to detect which subset of data a model is inaccurately predicting. Manifold also explains the potential cause of poor model performance by surfacing the feature distribution difference between better and worse-performing subsets of data.
Table of content
- Prepare your data
- Interpret visualizations
- Using Demo App
- Using the Component
- Contributing
- Versioning
- License
Prepare Your Data
There are 2 ways to input the data into Manifold:
- csv upload if you are using the Manifold demo app, or
- convert data programatically if you using the Manifold component in your own app.
In either case, data that's directly inputted into Manifold should follow this format:
const data = x: ... // feature data yPred: ... ... // prediction data yTrue: ... // ground truth data;
Each element in these arrays represents one data point in your evaluation dataset, and the order of data instances in x
, yPred
and yTrue
should all match.
Recommended instance count for each of these datasets is 10000 - 15000. If you have a larger dataset that you want to analyze, a random subset of your data generally suffices to reveal the important patterns in it.
x
: {Object[]}
A list of instances with features. Example (2 data instances):
feature_0: 21 feature_1: 'B' feature_0: 36 feature_1: 'A'
yPred
: {Object[][]}
A list of list, each child list is a prediction array from one model for each data instance. Example (3 models, 2 data instances, 2 classes ['false', 'true']
):
false: 01 true: 09 false: 08 true: 02 false: 03 true: 07 false: 09 true: 01 false: 06 true: 04 false: 04 true: 06
yTrue
: {Number[] | String[]}
A list, ground truth for each data instance. Values must be numbers for regression model, must be strings that match object keys in yPred
for classification models. Example (2 data instances, 2 classes ['false', 'true']):
'true' 'false'
Interpret visualizations
This guide explains how to interpret Manifold visualizations.
Manifold consists of:
- Performance Comparison View which compares prediction performance across models, across data subsets
- Feature Attribution View which visualizes feature distributions of data subsets with various performance levels
Performance Comparison View
This visualization is an overview of performance of your model(s) across different segments of your data. It helps you identify under-performing data subsets for further inspection.
Reading the chart
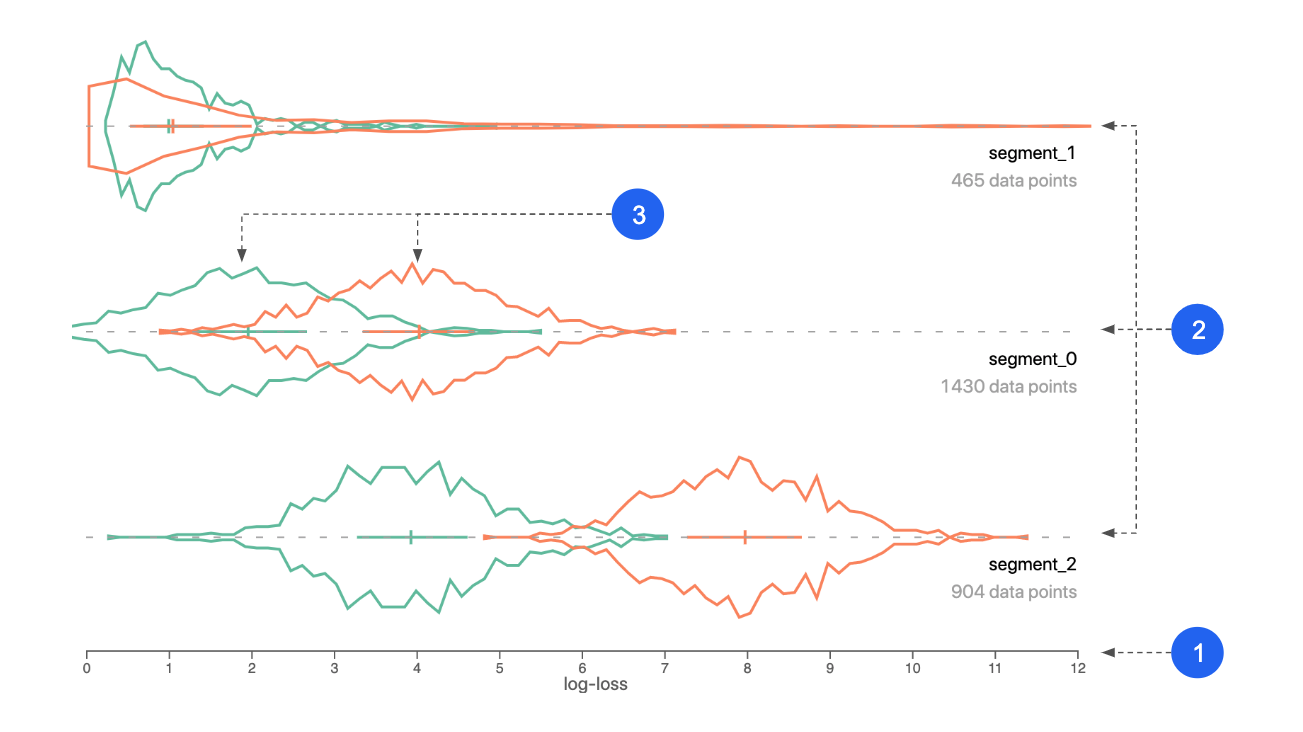
- X axis: performance metric. Could be log-loss, squared-error or raw prediction.
- Segments: your dataset is automatically divided into segments based on performance similarity between instances, across models.
- Colors: represent different models.
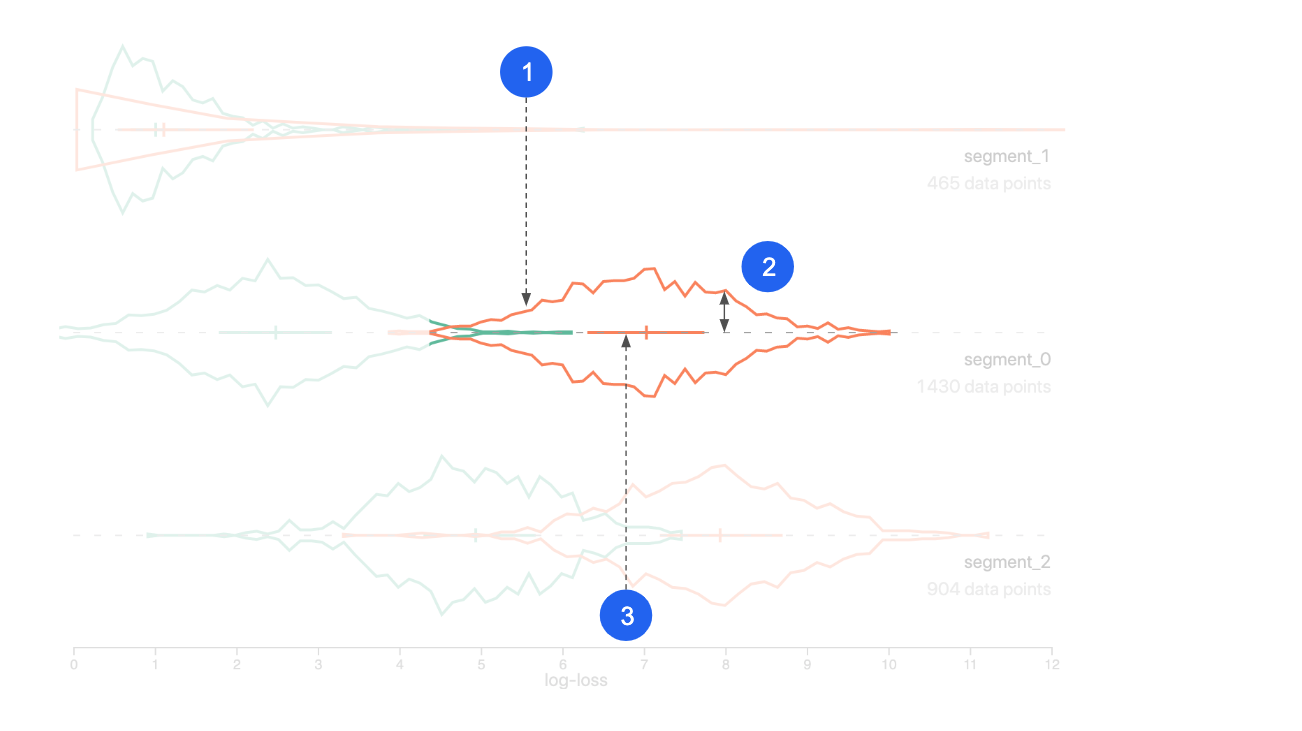
- Curve: performance distribution (of one model, for one segment).
- Y axis: Data count/density.
- Cross: left end, center line, and right end are 25, 50 and 75th percentile of the distribution.
Explaination
Manifold uses a clustering algorithm (k-Means) to break prediction data into N segments based on performance similarity.
The input of the k-Means is per-instance performance scores. By default, that is log-loss value for classification models and squared-error value for regression models. Models with a lower log-loss/squared-error perform better than models with a higher log-loss/squared-error.
If you're analyzing multiple models, all models' performance metrics will be included in the input.
Usage
-
Look for segments of data where error is higher (plotted to the right). These are areas you should analyze and try to improve.
-
If you're comparing models, look for segments where the log-loss is different for each model. If two models perform differently on the same set of data, consider using the better-performing model for that part of the data to boost performance.
-
After you notice any performance patterns/issues in the segments, slice the data to compare feature distribution for the data subset(s) of interest. You can create two segment groups to compare (colored pink and blue), and each group can have 1 or more segments.
Example
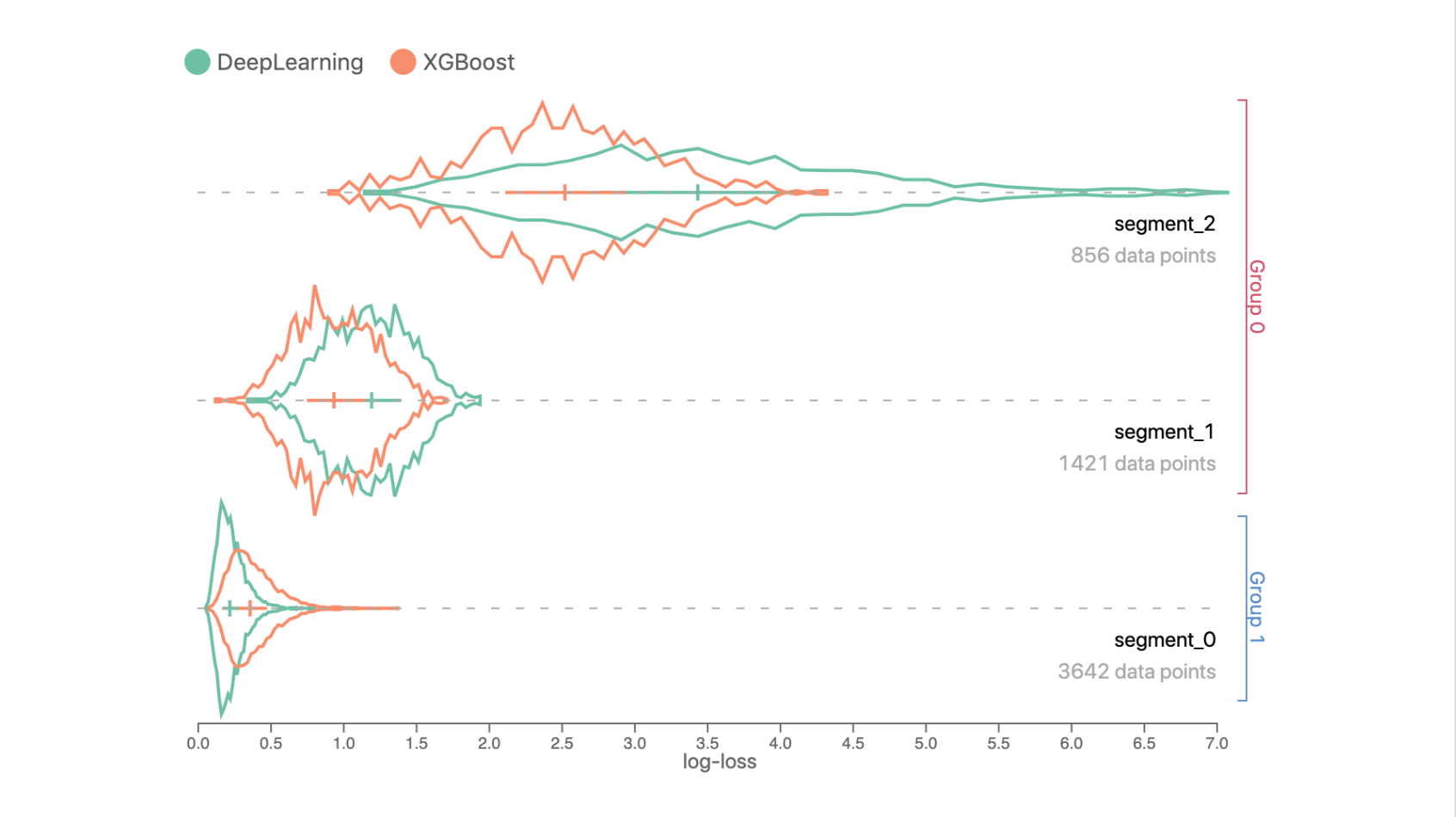
Data in Segment 0 has lower log-loss prediction error compared to Segments 1 and 2, since curves in Segment 0 is closer to the left side.
In Segments 1 and 2, the XGBoost model performs better than the DeepLearning model, but DeepLearning outperforms XGBoost in Segment 0.
Feature Attribution View
This visualization shows feature values of your data, aggregated by user-defined segments. It helps you identify any input feature distribution that might correlate with inaccurate prediction output.
Reading the chart
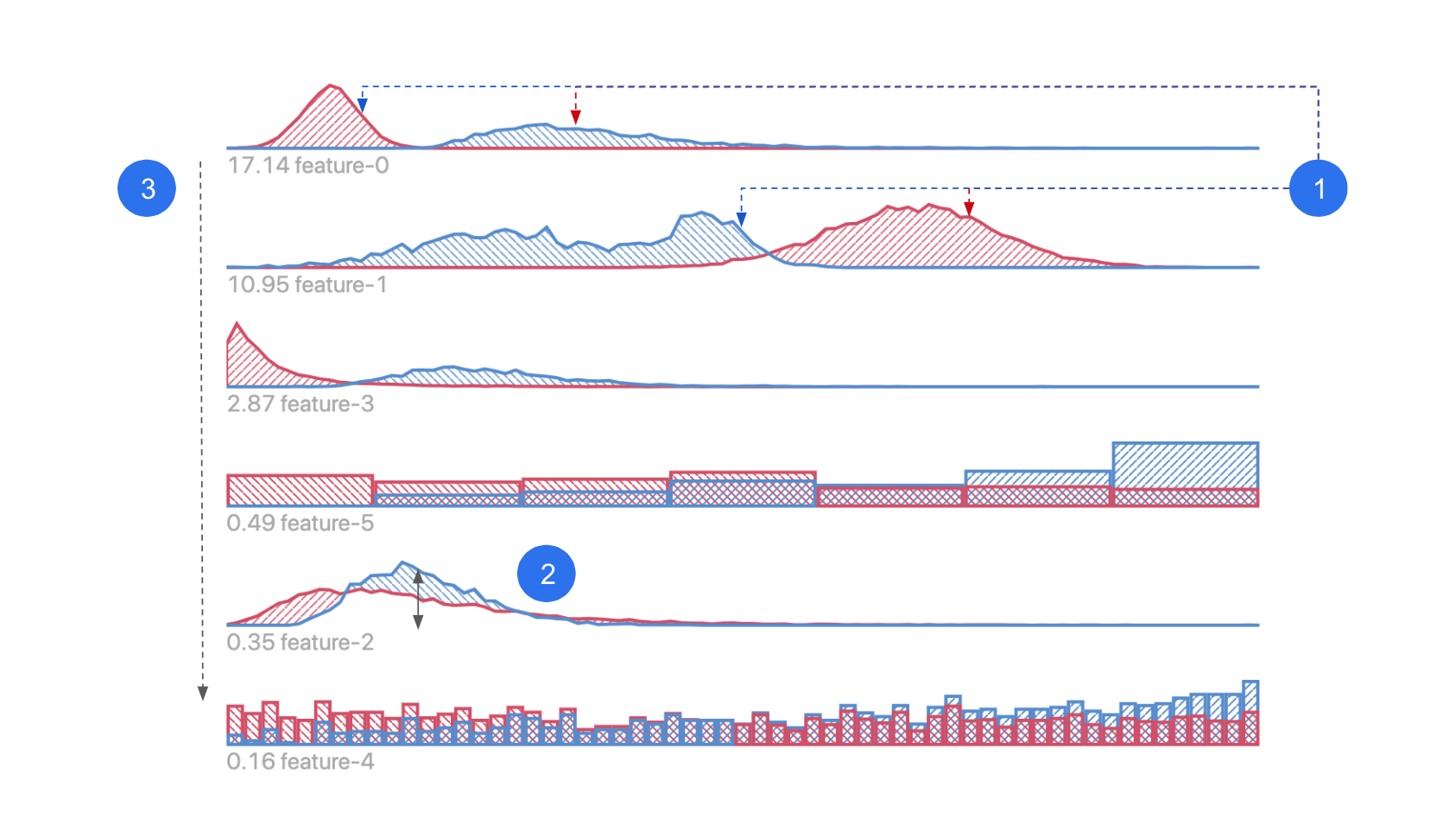
- Histogram / heatmap: distribution of data from each data slice, shown in corresponding color.
- Segment groups: indicates data slices you choose to compare against each other.
- Ranking: features are ranked by distribution difference between slices.
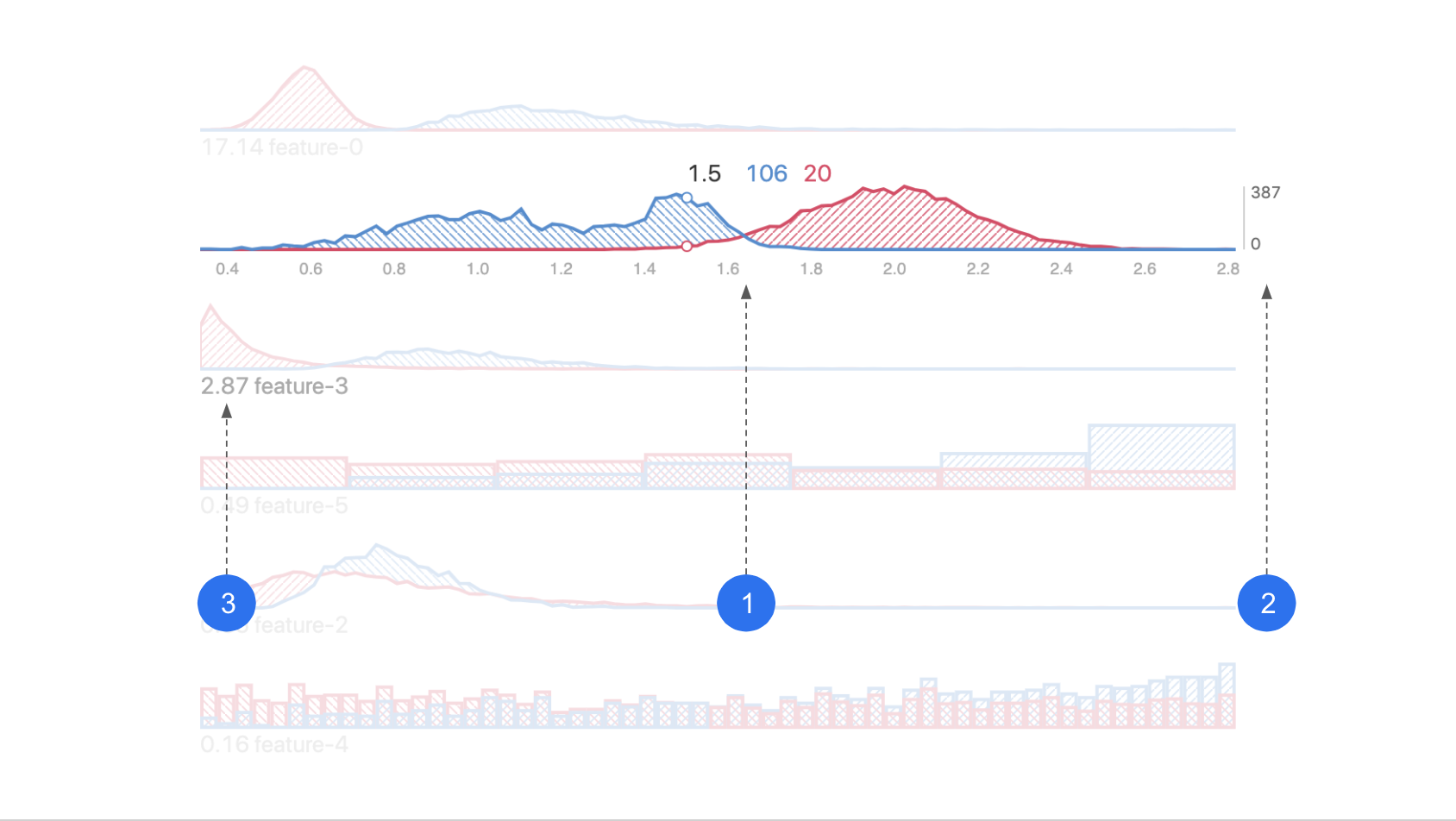
- X axis: feature value.
- Y axis: Data count/density.
- Divergence score: measure of difference in distributions between slices.
Explanation
After you slice the data to create segment groups, feature distribution histograms/heatmaps from the two segment groups are shown in this view.
Depending on the feature type, features can be shown as heatmaps on map for geo features, distribution curve for numerical features, or distribution bar chart for categorical features (In bar charts, categories on x-axis are sorted by instance count differenceLook for differences between the two distributions in each feature.)
Features are ranked by their KL-Divergence - a measure of difference between the two contrasting distributions. The higher the divergence is, the more likely this feature is correlated with the factor that differentiates the two Segment Groups.
Usage
- Look for the differences between the two distributions (pink and blue) in each feature. They represent the difference in data from the two segment groups you selected in the Performance Comparison View.
Example
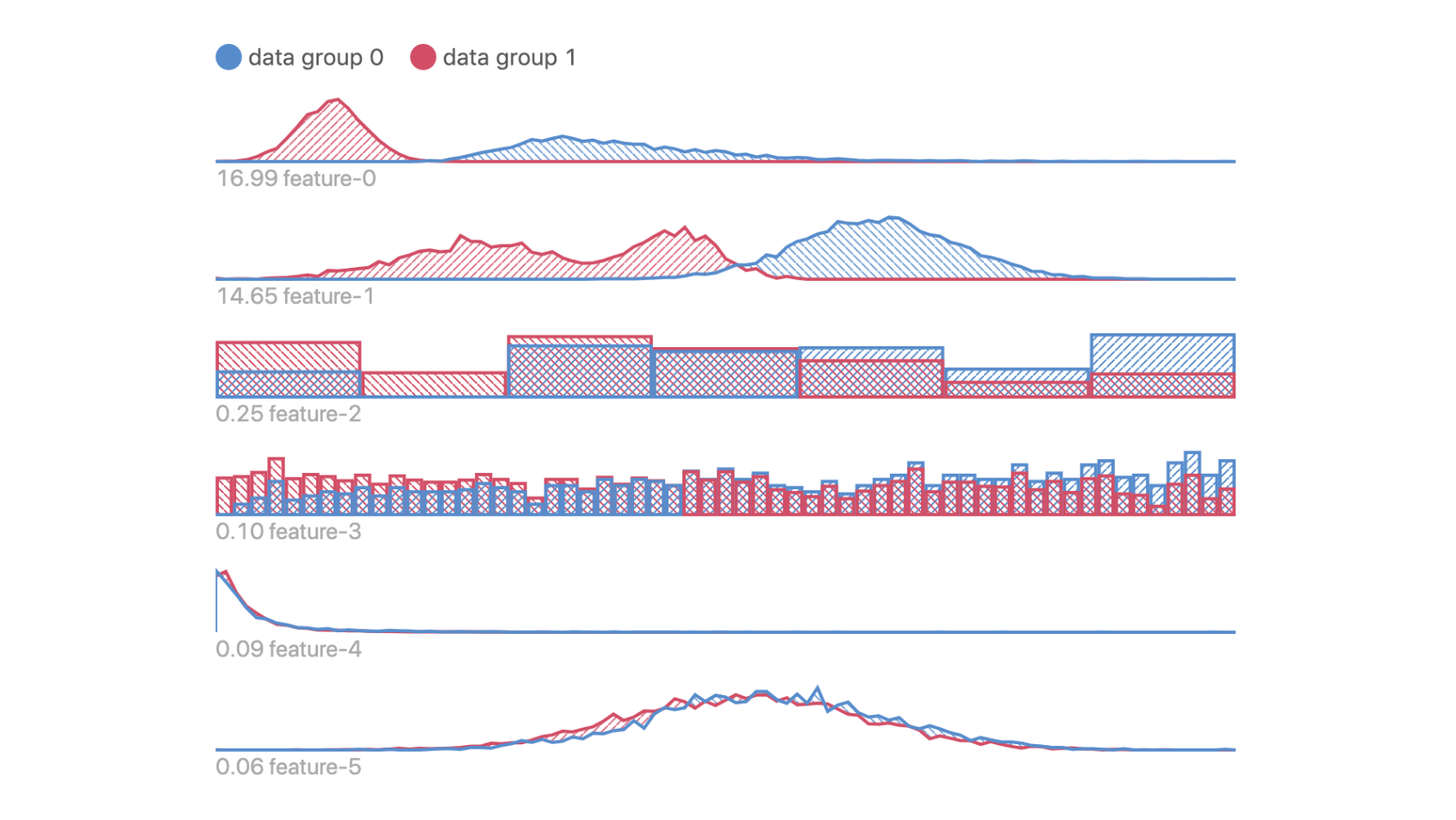
Data in Groups 0 and 1 have obvious difference in Features 0, 1, 2 and 3; but they are not so different in features 4 and 5.
Suppose Data Groups 0 and 1 correspond to data instances with low and high prediction error respectively, this means that data with higher errors tend to have lower feature values in Features 0 and 1, since peak of pink curve is to the left side of the blue curve.
Using Demo App
To do a one-off evaluation using static outputs of your ML models, using the demo app is an easier way for you. Otherwise, if you have a system that programmatically generates ML model outputs, you might consider using the Manifold component directly.
Running Demo App Locally
Run the following commands to set up env and run the demo:
# under the root directory, install all dependencies yarn# demo app is in examples/manifold directory cd examples/manifold# instal demo app dependencies yarn# start the app npm run start
Now you should see the demo app running at localhost:8080
.
Upload CSV to Demo App
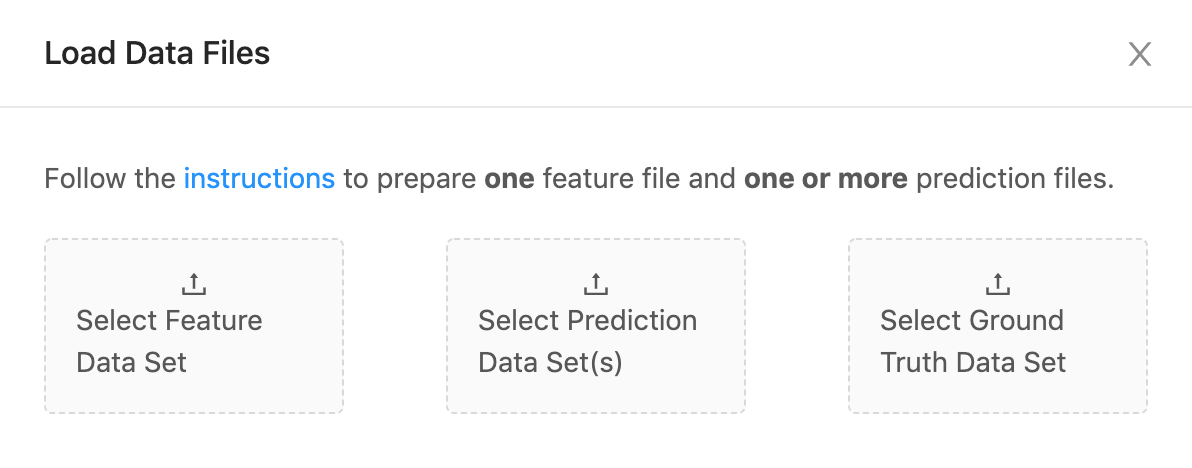
Once the app starts running, you will see the interface above asking for uploading "feature", "prediction" and "ground truth" datasets to Manifold.
They correspond to x
, yPred
, yTrue
in "prepare your data" section, and you should prepare your CSV files accordingly, illustrated below:
Field | x (feature) |
yPred (prediction) |
yTrue (ground truth) |
---|---|---|---|
Number of CSV's | 1 | multiple | 1 |
Illustration of CSV format | ![]() |
![]() |
![]() |
Once the datasets are uploaded, you will see visualizations generated by these datasets.
Using the Component
Embedding the Manifold component in your app allows you to programmatically generate ML model data and visualize. Ohterwise, if you have some static output from some models and want to do a one-off evaluation, you might consider using the demo app directly.
Here are the basic steps to import manifold into your app and load data for visualizing. You also take a look at the examples folder.
Install Manifold
$ npm install @uber/manifold
Load and Convert Data
In order to load your data files to Manifold, use loadLocalData
action. You could also reshape your data into the required Manifold format using dataTransformer
.
; // create the following action and pass to dispatch;
fileList
: {Object[]}
One or more datasets, in CSV format. Could be ones that your backend returns.
dataTransformer
: {Function}
A function that transforms fileList
into the Manifold input data format. Default:
const defaultDataTransformer = x: yPred: yTrue: ;
Mount reducer
Manifold uses Redux to manage its internal state. You need to register manifold reducer to the main reducer of your app:
;; const initialState = {};const reducers = ; // using createStorereducer initialState;
Mount Component
If you mount manifold reducer in another address instead of manifold
in the step above, you will need to specify the path to it when you mount the component
with the getState
prop.
; const Main = <Manifold getState= statepathTomanifold/>;
Built With
Contributing
Please read our code of conduct before you contribute! You can find details for submitting pull requests in the CONTRIBUTING.md file. Issue template.
Versioning
We document versions and changes in our changelog - see the CHANGELOG.md file for details.
License
Apache 2.0 License